IDRC AI4COVID Response Program
Project Title: Predictive modeling and forecasting of the transmission of COVID- 19 in Africa using Artificial Intelligence
Funders: IDRC and SIDA
Project Budget (in $ CAD): 1,249,030
The objective of the project was to develop and adapt existing models and simulations on COVID-19 transmission dynamics as well as public health interventions (PHIs), locally relevant to specific African urban and peri-urban environments to: (a) Develop modelling tools and simulation dashboards relevant and practical to local health authorities and policy-makers in mitigating or suppressing the impact of subsequent waves of infections; (b) Evaluate relative effectiveness and potential biases of PHIs (e.g. local appropriateness and feasibility, cost, socio-economic impact); and (c) Support communication strategies with local stakeholders that address dis- and mis-information about COVID-19 prevention and treatment.
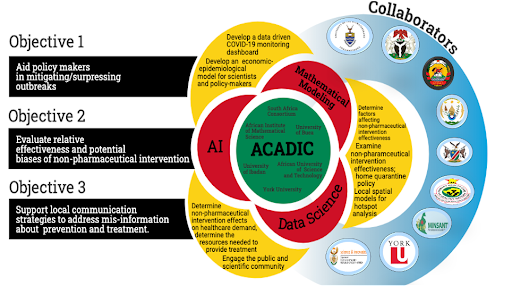
Achievements
ACADIC has successfully delivered locally nuanced analyses to monitor COVID-19, predict resurgences, identify and analyze emergent hotspots and outbreaks, identify individuals at higher risk of infection, stratify patients, identify gendered vulnerability, and develop strategic, highly targeted and staged delivery plans of vaccines to priority areas. We are also doing ongoing monitoring to enhance testing and development to ensure that public health interventions are equitable and effective. Our models are the official models used by governments in their interactions with both local and national policymakers (see attached file for a detailed summary of our achievements). This has been achieved with $1.25 M support from the IDRC and SIDA. This has allowed us to draw on the government related networks of our team leaders in Botswana, Eswatini, Cameroon, Mozambique, Namibia, Nigeria, Rwanda, South Africa and Zimbabwe. Many of our team leaders also lead their national COVID-19 advisory committee or modelling task force (e.g Professor Bruce Mellado is the Head of the Gauteng Province Premier’s COVID-19 Advisory Committee and head of Southern Africa COVID-19 Modelling Taskforce; Professor Wilfred Ndifon is one of the leaders of Rwanda’s COVID-19 Modelling Taskforce ; Professor Ngwa Gideon is the head of the South West province, Cameroon Modelling Taskforce ; Professor Jude Dzevela Kong, the lead PI is a member of the Africa CDC Modelling Taskforce). The experience of our team leaders, their community partners, their detailed understanding of national and local context and constraints and opportunities, have each been core to the success-to-date of the ACADIC Consortium.
Below are some of the items we have successfully delivered and continue to deliver:
- Monitoring and forecasting the growth and spread of COVID-19 at the local, state, and national levels
- Evaluating efforts to mitigate and control the spread
- Identifying trends in the disease infections, hospitalizations, and deaths
- Guiding purchase and allocation of health care resources
- Guiding the collection of data (ensuring that data disaggregated by race, gender, sexuality, class, geographic location and Indigeneity)
- Assessing the impact of different vaccine distribution strategies
- Providing situational intelligence: on populations at risk, stage of outbreak, projected burden of illness, school/business/work closure and re-opening etc.
- Nowcasting labor market flow
- Supporting race, gender, sexuality, class, geographic location and Indigeneity, inclusive COVID-19 actions.
- Developing methodologies and technologies to describe contact mixing and transmission networks to quantify impacts of contact shifting and individual mobility
- Supporting transparent and responsible AI, data, and digital rights governance around COVID-19 and pandemic responses
- Strengthening data systems and information sharing about COVID-19
- Building trust and combatting mis- and dis-information around COVID-19
- Optimizing public health system responses for patient diagnosis, care, and management.
- Establishing sustainable collaborations among model developers, policy makers, community leaders etc
- Preparing the next generation of leaders in infectious disease modelling approaches in these countries
- Working closely with public health agencies and other stakeholders to build trust and knowledge of artificial intelligence models among key decision makers
- Developing stand-alone and predictive public health decision support tools.
- Creating a collaborative network that can respond rapidly to support decision makers in each country to address infectious diseases or other disasters and emergency situations in general.
In addition to the reports in the supplementary material, below are links to examples of the reports that we submit to the governments in every country (using South Africa as an example):
- https://www.dropbox.com/s/bea9yo8fw3b3gph/COVID19_PCCC_301121.ppt?dl=0
- https://www.dropbox.com/s/xneylee8glctdha/COVID19_PCCC_110122.ppt?dl=0
- https://www.dropbox.com/s/kwsihpur40kno2r/COVID19_PCCC_141221.ppt?dl=0
- https://www.dropbox.com/s/vmc1hrzjelbmvdh/COVID19_PCCC_161121.ppt?dl=0
- https://www.dropbox.com/s/57rbzuk5g7gy3pf/COVID19_PCCC_091121.ppt?dl=0
- https://www.dropbox.com/s/rd7viz2lsqgmvs0/COVID19_PCCC_280921.ppt?dl=0
- https://www.dropbox.com/s/0x8afnned2e4g2v/COVID19_PCCC_210921.ppt?dl=0
- https://www.dropbox.com/s/hkmvdmhqw86z0hp/COVID19_PCCC_140921.ppt?dl=0
- https://www.dropbox.com/s/yyamlx5tjlb4580/COVID19_PCCC_070921.ppt?dl=0
- https://www.dropbox.com/s/i4qankdst16675x/COVID19_PCCC_310821.ppt?dl=0
- https://www.dropbox.com/s/qsfeu3kr03bgjnm/COVID19_PCCC_240824.ppt?dl=0
- https://www.dropbox.com/s/fxovb7iqplnqs7o/COVID19_PCCC_170821.ppt?dl=0
- https://www.dropbox.com/s/x71rhxcn5fief2y/COVID19_PCCC_100821.ppt?dl=0
- https://www.dropbox.com/s/y4mnhuurh0dikwq/COVID19_PCCC_030821.ppt?dl=0
- https://www.dropbox.com/s/y4h77degmhouqp8/COVID19_PCCC_270721.ppt?dl=0
Research Findings
By drawing on the connections our team leaders in Botswana, Eswatini, Cameroon, Mozambique, Namibia, Nigeria, Rwanda, South Africa and Zimbabwe have with the governments of these countries; the fact that they are heads of COVID-19 advisory committee or modelling task force; their set of experiences; their community partners; local context; constraints; and possibilities of each country’ team etc., we have successfully delivered locally nuanced analyses to monitor COVID-19, predict resurgences, identify and analyze emergent hotspots and outbreaks, identify individuals at higher risk of infection, stratify patients, identify gendered vulnerability, and developed strategic, highly targeted and staged delivery plans of vaccines to priority areas.
We are also doing ongoing monitoring to enhance testing and development to ensure public health interventions are equitable and effective. Our models are the official models used by governments in their interactions with both local and national policymakers. A video summary of our achievements can be found here: https://youtu.be/kplM7hMy5y0. Below we have outlined some of the achievements for the first year.
1. We have developed COVID-19 monitoring dashboards that visualize locally relevant information to the public and policy makers: http://acadic.org/
These dashboards are the official dashboards used by policy makers in Botswana, Eswatini, Cameroon,
Mozambique, Namibia, Nigeria, Rwanda, South Africa and Zimbabwe. The website for South Africa is viewed by more than one million people daily and those for the other countries is viewed by more 50 thousand people daily. Below is a link to the Dashboards
- South Africa: http://www.covid19sa.org/
- Botswana: https://tinyurl.com/4e83hubd
- Eswatini: https://tinyurl.com/2p9eyeff
- Mozambique: https://tinyurl.com/3wrxwvr7
- Cameroon: https://www.yorku.ca/science/mathstats/acadic/cameroon/
- Nigeria: https://tinyurl.com/ytmxuzmp
A disease outbreak response requires timely exchange of information between policy makers and the public.
People are usually keen to see how the dynamics is evolving in their communities where their families live, or places they plan to travel to. This dictates the need for an urgent dashboard during an outbreak. To this end we integrated the power of Artificial Intelligence, predictive modelling, and simulations, to develop COVID-19 monitoring dashboards that visualize information locally relevant to the public and policy makers.
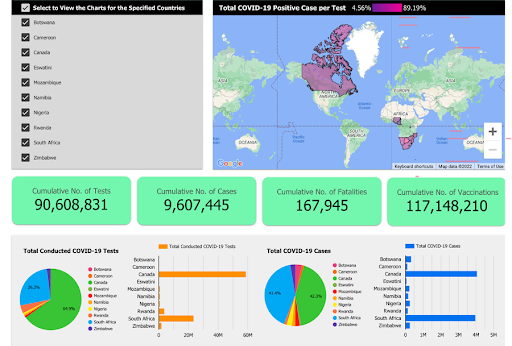
2. Obtaining locally relevant data in Africa to train AI algorithms. Obtaining locally relevant data in Africa to train AI-based algorithms is essential to inform public health policies in an evidence- and data-driven fashion. However, in Africa, this is rather challenging. During an outbreak, community leaders are usually aware of what is happening in the local community, and modelers have the tools to solve the problem but most often these people do not talk to each other. ACADIC team includes community leaders (e.g., chiefs, fons etc), and policy makers. The collaboration among ACADIC multi-disciplinary and multi- stakeholder team of scientists, mathematicians, public health experts, and community leaders has been helpful in securing locally relevant data. In ACADIC the community leaders articulate the problem in their communities, and the modelers inform the collection of data for the problem. The community is involved at every step of the process and the models are tailor-built to solve real-life problems affecting the community. This has been one of the keys to our success in informing COVID-19 policies in Africa.
3. Providing governments with a precise portrait of the epidermic at the local, state, and national levels-using a hybrid of Mechanistic and AI models. In Africa, there are stigmas attached to being infected with SARS-CoV-2. Moreover, self-medication and the use of complementary/alternative medicine are common among Africans for social, economic, and psychological reasons. Governments in these countries are generally faced with a number ofchallenges, including limited resources and poor infrastructure and patient’s health literacy. For COVID-19, this is fueled by the rapid spread of rumours in favour of these modalities on social media. Hence, irrespective of how ACADIC/public health authorities are with the communities, there is bound to be so much under-reported data in most communities. Thus, to have a clear picture of the nature of an epidermic (true case count, basic reproduction number, relative infectivity of the severe infectious, mild infectious and asymptomatic), after securing relevant data, ACADIC modelers design AI based algorithm to estimate under reported values.
Using AI and Big Data to Inform decisions about non-pharmaceutical Interventions during COVID-19. Now with the capability to generate true case count, we next design different indices for each local community, using the true case count and other locally relevant information. Using this data, we trained an AI algorithm to come up with a 5-level alert system by splitting the jump from full-lockdown back to business as usual into 5 levels. In order to quantify the effects of the alert system, a Stringency Index was carefully created for each Alert Level. The calculation of this Stringency index takes into account the status of various indicators that represent a number of Non-Pharmaceutical Interventions.
3. Using AI and Big Data techniques to provide predictions of future COVID-19 peaks and of their intensities. During the COVID-19, ACADIC has been able to predict all the waves in each community in countries across Africa. The figure below shows the third wave predictions in South Africa by ACADIC.
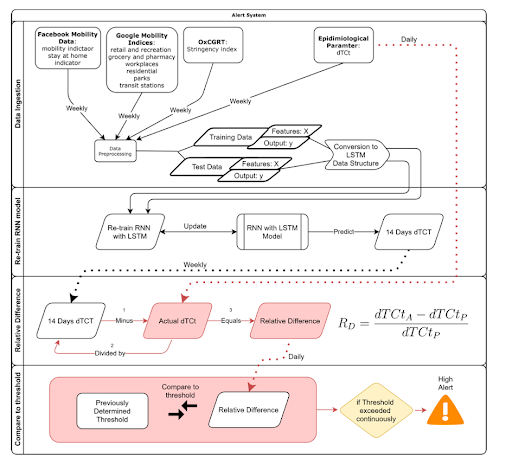
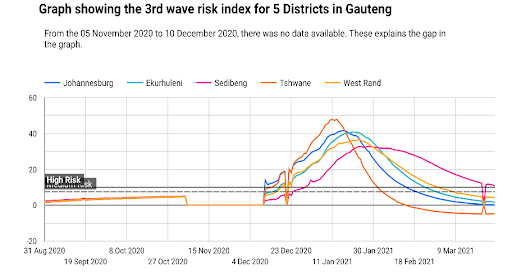
6. Using AI and Big Data techniques to identify COVID-19 Hotspots:
The importance of hotspots also known as spatial clusters in infectious disease epidemiology cannot be over emphasized. Identifying local hotspots for an epidermic help to inform resource allocation and programmatic goals. Over the past decade there has been increasing interest in spatial clusters for infectious diseases. This includes calls to target spatial clusters in an outbreak. For example, recommendations to focus on hotspots has been part of strategic plans for Ebola, malaria, COVID-19 and other emerging pandemic threats. In the ongoing COVID-19, ACADIC, visualizes hotspots in each local community across Africa and this have been helpful to policymakers at different levels and the population.
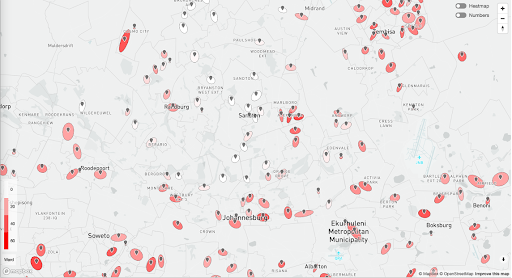
7. Leveraging AI and Big Data techniques to optimize clinical public health and vaccination roll-out strategies.
Once vaccine became available in Africa, ACADIC leveraged AI and Big Data techniques to develop strategic, highly targeted and staged delivery plans of vaccines to prioritize vulnerable, at-risk population and hotspots areas. Deploying and administering effective and safe vaccines against a novel disease to large populations at a fast pace is a nontrivial task. Due to the logistical challenges that exist in Africa, an assessment of different vaccination scenarios and roll-out strategies, that take into account national and local realities, is of paramount importance for public health policy and decision making, in order to maximize and track staged progress towards the achievement of herd immunity.
8. Providing a near term forecasts (nowcasting) of labour market flows in Africa during the pandemic:
The unemployment rate is an important economic indicator that is input into decision making by policy makers. In Africa, they are usually released infrequently with substantial delay. The speed of economic decline during the onset of COVID-19 made policy makers confused as to what to do to get timely information about the labour market. To this end, ACADIC immediately employ artificial intelligence to provide a framework that can be used to provide more timely information about the unemployment rate in countries across Africa. In the upcoming year, there will also be uncertainty about the timing and extent of any improvement in labour market outcomes that will also highlight the value of the framework that ACADIC came up with.
9. Monitoring social distancing on University campuses
We design an AI powered toll-kit for measuring social distance in universities. This toll-kit is currently being used at Witwatersrand University and other Universities across South Africa.
10. Address dis- and mis-information about COVID-19 prevention, treatment and vaccines:
Since the outbreak of COVID-19, rumours about COVID-19 have been doing rounds on social media in Africa. These include: i) “this or that ” tradition medicine can cure COVID- 19 or prevent one from having it ii) “afofo” (a locally made alcohol with an unknown alcoholic content) can prevent you from having COVID iii) “’this or that pastor” cures COVID-19 iv) SARS-CoV-2 does not affect Africans, iv) chloroquine cures COVID-19, iv) spraying alcohol and chlorine all over your body will prevent you from having COVID v) SARS-CoV-2 cannot survive in Africa’s warm climate vi) steaming your face with and inhale neem tree leaves prevent you from having COVID-19 vii) vitamin C tablets prevent COVID- 19 viii) having had malaria makes one immune, ix) pepper soup with lime or lemon flushes out the virus x) drinking black tea first thing in the morning prevents you from having COVID-19. To support local stakeholders in addressing this infodemic, ACADIC employed artificial intelligence and big data to identify the hot spots of infodemic. In addition, ACADIC helped support communication strategies with local stakeholders that addresses the root courses of this infodemic.
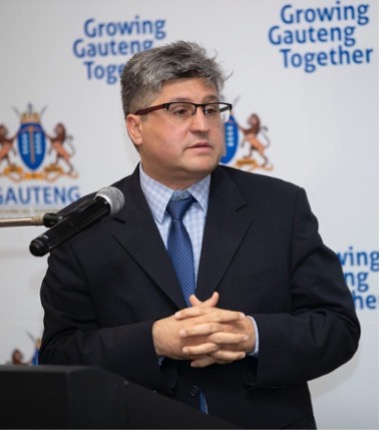
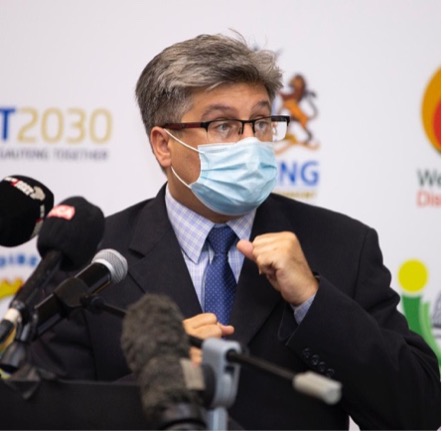
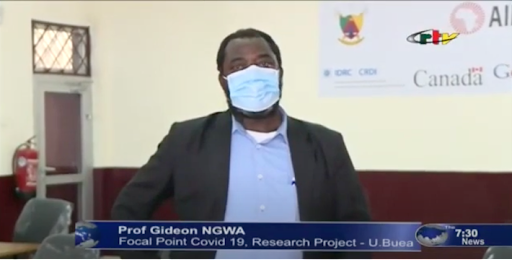
11. Media Coverage
Our work has been covered on all main TV, Radio and newspapers of these countries and other international medias hundreds of times. A selection of news bits can be found here. Also, below you will find a link to some of the media coverage
- https://www.thestar.com/local-toronto-north-york/news/2021/08/12/york-university- leads-team-that-uses-artificial-intelligence-to-fight-covid-19-in-africa.html
- https://www.dw.com/en/covid-artificial-intelligence-in-the-pandemic/a-58171146
- https://youtu.be/4xN8JrBN5XA
- https://youtu.be/Sm_J5WX_di8
- https://youtu.be/4H6iiPRmtW0
- https://youtu.be/5AM9h0y5ptI
- https://tinyurl.com/2c9a2ajn
- https://toronto.ctvnews.ca/misinformation-on-social-media-linked-to-higher-spread-
of-covid-19-in-new-study-1.5466846 - https://www.theregister.com/2021/06/11/pandemic_drinking_and_swearing_outbreak/
- https://phys.org/news/2021-06-social-media-factors-higher-covid-.html
- https://www.eurekalert.org/news-releases/617830
- https://tinyurl.com/3zbf6ht9
- https://www.fairchildtv.com/newsarchive_detail.php?n=28
- https://www.ctvnews.ca/video?clipId=2401509
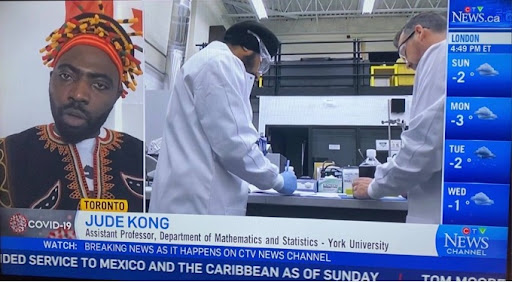
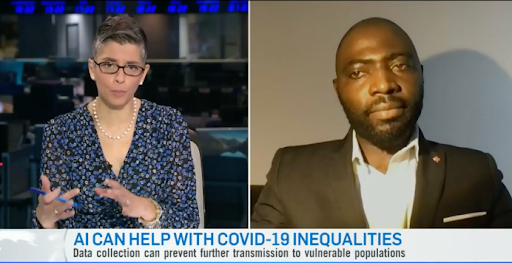
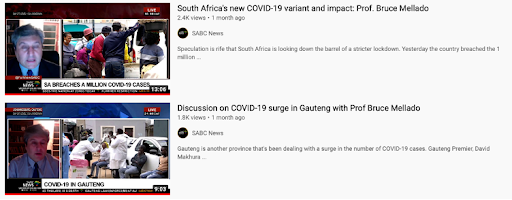
Our team leaders are regularly invited by governments to participate in the press conferences given to the population to raise awareness of where we are in the pandemic and what measures need to be emphasized at any given time.
12. Conference/workshops/webinar series/presentations.
We have given over 200 presentations within the first year to different work streams in the government. Most of these presentations are given during closed doors sessions and cannot be shared here. Below is a link to sample reports that the team share with policy makers.
- https://www.dropbox.com/s/bea9yo8fw3b3gph/COVID19_PCCC_301121.ppt?dl=0
- https://www.dropbox.com/s/xneylee8glctdha/COVID19_PCCC_110122.ppt?dl=0
- https://www.dropbox.com/s/kwsihpur40kno2r/COVID19_PCCC_141221.ppt?dl=0
- https://www.dropbox.com/s/vmc1hrzjelbmvdh/COVID19_PCCC_161121.ppt?dl=0
- https://www.dropbox.com/s/57rbzuk5g7gy3pf/COVID19_PCCC_091121.ppt?dl=0
- https://www.dropbox.com/s/rd7viz2lsqgmvs0/COVID19_PCCC_280921.ppt?dl=0
- https://www.dropbox.com/s/0x8afnned2e4g2v/COVID19_PCCC_210921.ppt?dl=0
- https://www.dropbox.com/s/hkmvdmhqw86z0hp/COVID19_PCCC_140921.ppt?dl=0
- https://www.dropbox.com/s/yyamlx5tjlb4580/COVID19_PCCC_070921.ppt?dl=0
- https://www.dropbox.com/s/i4qankdst16675x/COVID19_PCCC_310821.ppt?dl=0
- https://www.dropbox.com/s/qsfeu3kr03bgjnm/COVID19_PCCC_240824.ppt?dl=0
- https://www.dropbox.com/s/fxovb7iqplnqs7o/COVID19_PCCC_170821.ppt?dl=0
- https://www.dropbox.com/s/x71rhxcn5fief2y/COVID19_PCCC_100821.ppt?dl=0
- https://www.dropbox.com/s/y4mnhuurh0dikwq/COVID19_PCCC_030821.ppt?dl=0
- https://www.dropbox.com/s/y4h77degmhouqp8/COVID19_PCCC_270721.ppt?dl=0
We have also given over 100 presentations in national and international conferences/seminars. Each country runs a weekly webinar series where they invite researchers from around the world to come and share their research work with them. We meet bi-weekly for updates from each country, and this has been going on since we started working on the project.
13. Publications
The consortium has published several peer-reviewed articles as well have several articles currently being reviewed. Below is a list of some of the where some of our findings can be found.
1)Stevenson, F., Hayasi, K., Bragazzi, N. L., Kong, J. D., Asgary, A., Lieberman, B., … & Wu, J. (2021). Development of an Early Alert System for an Additional Wave of COVID-19 Cases Using a Recurrent Neural Network with Long Short-Term Memory. International Journal of Environmental Research and Public Health, 18(14), 7376.
2) Kong, J. D., Tchuendom, R. F., Adeleye, S. A., David, J. F., Admasu, F. S., Bakare, E. A., & Siewe, N. (2021). SARS-CoV-2 and self-medication in Cameroon: a mathematical model. Journal of Biological Dynamics, 15(1), 137-150.
3)Mellado, B., Wu, J., Kong, J. D., Bragazzi, N. L., Asgary, A., Kawonga, M., … & Orbinski, J. (2021). Leveraging artificial intelligence and big data to optimize COVID-19 clinical public health and vaccination roll-out strategies in Africa. International Journal of Environmental Research and Public Health, 18(15), 7890.
4)Potgieter, A., Fabris-Rotelli, I. N., Kimmie, Z., Dudeni-Tlhone, N., Holloway, J. P., van Rensburg, C. J., … & Khuluse-Makhanya, S. (2021). Modelling representative population mobility for COVID-19 spatial transmission in South Africa. Frontiers in big Data, 4.
5)Duhon, J., Bragazzi, N., & Kong, J. D. (2021). The impact of non-pharmaceutical interventions, demographic, social, and climatic factors on the initial growth rate of COVID-19: A cross-country study. Science of The Total Environment, 760, 144325.
6)Kong, J. D., Tekwa, E. W., & Gignoux-Wolfsohn, S. A. (2021). Social, economic, and environmental factors influencing the basic reproduction number of COVID-19 across countries. PloS one, 16(6), e0252373.
7)Tao, S., Bragazzi, N. L., Wu, J., Mellado, B., & Kong, J. D. (2022). Harnessing Artificial Intelligence to assess the impact of nonpharmaceutical interventions on the second wave of the Coronavirus Disease 2019 pandemic across the world. Scientific reports, 12(1), 1- 9.
8) Lieberman, B., Gusinow, R., Asgary, A., Bragazzi, N. L., Choma, N., Dahbi, S. E., … & Wu, J. (2021). Big Data-and Artificial Intelligence-Based Hot-Spot Analysis of COVID- 19: Gauteng, South Africa, as a case study. South Africa, as a case study (March 13, 2021).
9) Bouba Y, Tsinda EK, Fonkou MDM, Mmbando GS, Bragazzi NL, Kong JD. The Determinants of the Low COVID-19 Transmission and Mortality Rates in Africa: A Cross-Country Analysis. Front Public Health. 2021 Oct 21;9:751197. doi:10.3389/fpubh.2021.751197. PMID: 34746085; PMCID: PMC8568130.
10) Iyaniwura, S. A., Musa, M. R., David, J. F., & Kong, J. D. (2021). The basic reproduction number of COVID-19 across Africa. medRxiv.
11) Mbogning Fonkou, M. D., Bragazzi, N. L., Tsinda, E. K., Bouba, Y., Mmbando, G. S., & Kong, J. D. (2021). Covid-19 pandemic related research in africa: Bibliometric analysis of scholarly output, collaborations and scientific leadership. International journal of environmental research and public health, 18(14), 7273.
12) Iyaniwura SA, Rabiu M, David JF, Kong JD. Assessing the impact of adherence to non- pharmaceutical interventions and indirect transmission on the dynamics of COVID-19: a mathematical modelling study. Math Biosci Eng. 2021 Oct 15;18(6):8905-8932. doi: 10.3934/mbe.2021439. PMID: 34814328.
13) Nia ZM, Asgary A, Bragazzi N, Melado B, Orbinski J, Wu J, Kong JD. Being Unemployed in South Africa during the COVID-19 Pandemic: Quantitative and Qualitative insights from Twitter Data. A Data- and Text-Mining Analysis. JMIR Preprints. 26/09/2021:33843
14) Kazemi, M., Bragazzi, N. L., & Kong, J. D. (2022). Assessing Inequities in COVID-19 Vaccine Roll-Out Strategy Programs: A Cross-Country Study Using a Machine Learning Approach. Vaccines, 10(2), 194.
15) Nia, Z., Ahmadi, A., Bragazzi, N. L., Woldegerima, W. A., Mellado, B., Wu, J., … & Kong, J. D. (2022). A Cross-Country Analysis of Macroeconomic Responses to COVID- 19 Pandemic Using Twitter Sentiments. Available at SSRN 4001976.
16) Yan, C., Law, M., Nguyen, S., Cheung, J., & Kong, J. (2021). Comparing Public Sentiment Toward COVID-19 Vaccines Across Canadian Cities: Analysis of Comments on Reddit. Journal of medical Internet research, 23(9), e32685.
17) Avusuglo, W., Bragazzi, N. L., Asgary, A., Orbinski, J., Wu, J., & Kong, J. D. (2021). The Role of Behavioral Compliance to Non-Pharmaceutical and Pharmaceutical Interventions in the Fight Against COVID-19: Insights from a Behavior-Disease Economic Epidemic Model Coupled With Optimal Control Theory. Available at SSRN 3922118.
18) Guelmami, N., Chalghaf, N., Wu, J., Kong, J. D., Mellado, B., Jahrami, H., … & Bragazzi, N. L. (2021). Social Media COVID-19 Information and Vaccine Decision: A Latent Class Analysis. Available at SSRN 3841301.
19) Fabris-Rotelli, I., Holloway, J., Kimmie, Z., Archibald, S., Debba, P., Docrat, R., … & Makhanya, S. (2021). A Spatial SEIR Model for COVID-19 in South Africa.
20) Thiede, R., Abdelatif, N., Fabris-Rotelli, I., Manjoo-Docrat, R., Holloway, J., van Rensburg, C. J., … & Roux, A. L. (2020). Spatial variation in the basic reproduction number of COVID-19: A systematic review. arXiv preprint arXiv:2012.06301.
21) Guelmami, N., Ben Khalifa, M., Chalghaf, N., Kong, J.D., Amayra, T., Wu, J., Azaiez, F. and Bragazzi, N.L., 2021. Preliminary development of the social media disinformation scale (SMDS-12) and its association with social media addiction and mental health: COVID-19 as a pilot case study. JMIR Formative Research, 5(6), p.e27280.
22) Kaur, M., Bragazzi, N. L., Heffernan, J. M., Tsasis, P., Wu, J., & Kong, J. D. (2021). COVID-19 in Ontario Long-term Care Facilities Project, a Manually Curated and Validated Database. Available at SSRN 3981025.
23) Cheong, Q., Au-Yeung, M., Quon, S., Concepcion, K., & Kong, J. D. (2021). Predictive Modeling of Vaccination Uptake in US Counties: A Machine Learning–Based Approach. Journal of medical Internet research, 23(11), e33231.
24) Ogbuokiri B, Ahmadi A, Bragazzi N, Nia Z, Mellado B, Wu J, Orbinski J, Asgary A, Kong J. On Public Sentiments Towards COVID–19 Vaccines in South African Cities: An Analysis of Twitter Posts. JMIR Preprints. 02/02/2022:36796
14. Building Capacity
Through our collaboration, we have built capacities for the use of artificial intelligence in public health in the various countries. Our highly qualified personnel meet weekly to learn from each other in addition to the meetings with their individual team leaders. Through the unique training that we are giving them, they have gained essential and transferable skills, including the capacity to develop research questions, design and analyze artificial intelligence models, manage data, mentor junior scientists, and conduct complex cutting -edge public health data analyses on large datasets using artificial intelligence and big data techniques. This has resulted to a critical mass of next generation world-class researchers and practitioners that will ensure these countries can mobilize the required expertise for modeling assisted rapid responses to existing and emerging public health issues. Each country can now boost of at least 5 experts in using artificial intelligence to address public health issues.